What is data reduction?
Data reduction methods can be used to lessen the amount of data that is physically stored. This saves storage space and costs.
What does data reduction mean?
The term data reduction covers various methods used to optimise capacity. Such methods aim to reduce the amount of data being stored. With data volumes increasing worldwide, data reduction is necessary to ensure resource- and cost-efficiency when storing data.
Data reduction can be carried out through data compression and deduplication. While lossless compression uses redundancies within a file to compress data, deduplication algorithms match data across files to avoid repetition.
What is deduplication?
Deduplication is a process of data reduction that is essentially based on preventing data redundancies in the storage system. It can be implemented either at the storage target or at the data source. A deduplication engine is used, which uses special algorithms to identify and eliminate redundant files or data blocks. The main area of application for deduplication is data backup.
The aim of data reduction using deduplication is to write only as much information on non-volatile storage media as is necessary to be able to reconstruct a file without losses. The more duplicates are deleted, the smaller the data volume that needs to be stored or transferred.
The identification of duplicates can be done at file-level with Git or Dropbox, for example. However, a more efficient method is the use of deduplication algorithms, which work on a sub-file level. To do this, files are first broken down into data blocks (chunks) and awarded unique checksums, or hash values. The tracking database, which contains every checksum, acts as a central supervisory entity.
The block-based deduplication methods can be broken down into two variations:
- Fixed block length: Files are divided into sections of exactly the same length based on the cluster size of the file or RAID system (typically 4 KB)
- Variable block length: The algorithm divides the data into different blocks, the length of which varies depending on the type of data to be processed.
The way blocks are divided has a massive influence on the efficiency of the data duplication. This is especially noticeable when deduplicated files are subsequently modified. When using fixed block sizes, if a file is changed, all subsequent segments are also classified as new by the deduplication algorithm due to the shift in block boundaries. This increases the computing effort and use of bandwidth.
If, on the other hand, an algorithm uses variable block boundaries, the modifications of an individual data block have no effect on the next segments. Instead, the modified data block is simply extended and stored with the new bytes. This relieves the burden on the network. However, the flexibility of the file changes is more computing-intensive, as the algorithm must first find out how the chunks are split up.
- Automatic backup and easy recovery
- Intuitive scheduling and management
- AI-based threat protection
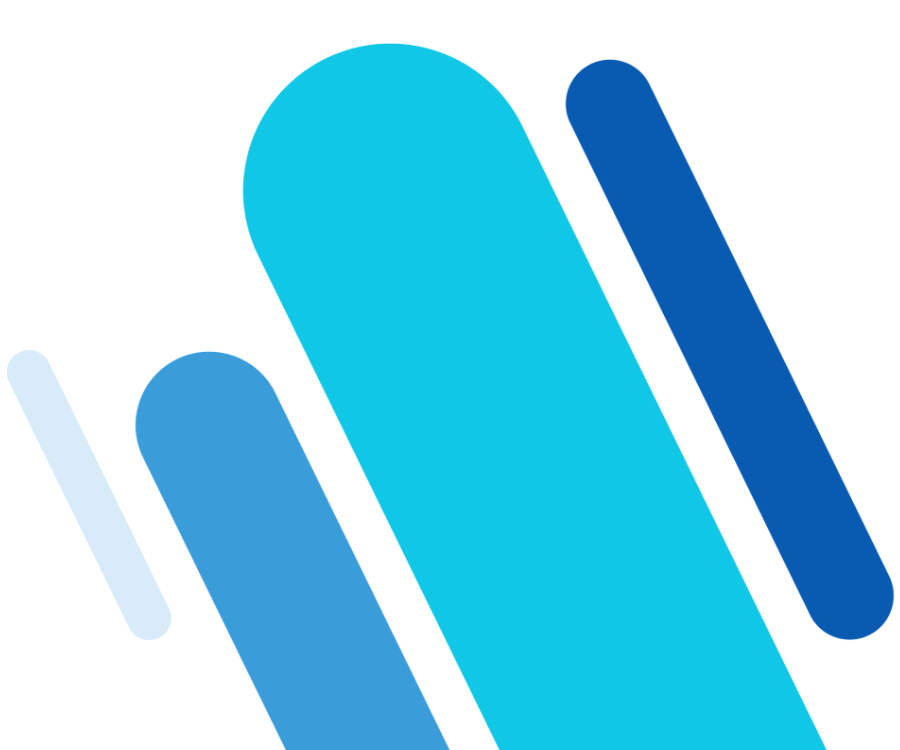
What is data compression?
In data compression, files are converted into an alternative format, which is more efficient than the original. The aim of this type of data reduction is to reduce the required memory space as well as the transfer time. A coding gain like this can be achieved with two different approaches:
- Redundancy compression: With lossless data compression, data can be decompressed precisely after compression. Input and output data is therefore identical. This kind of compression is only possible when a file contains redundant information.
- Irrelevance compression: With lossy compression, irrelevant information is deleted to compress a file. This is always accompanied by a loss of data. There is only an approximate recovery of the original data after an irrelevance compression. The process for classifying data as irrelevant is discretionary. In an audio compression via MP3, for example, the frequency patterns removed are those that are assumed to be hardly or not at all heard by humans.
While compression on the storage system level is essentially loss-free, data losses in other areas, such as image, video and audio transfers, are deliberately accepted to reduce file size.
Both the encoding and decoding of a file require computational effort. This primarily depends on the compression method that is used. While some techniques aim for the most compact representation of the original data, others focus on reducing the required computation time. The choice of compression method is therefore always dependent on the requirements of the project or task it is being used for.
Which data reduction method is better?
In order to implement backup procedures or optimise storage in standard file systems, companies generally rely on deduplication. This is mainly due to the fact that deduplication systems are extremely efficient when identical files need to be stored.
Data compression methods, on the other hand, are generally associated with higher computing costs and therefore require more complex platforms. Storage systems that have a combination of both data reduction methods can be used most effectively. First, redundancies are removed from the files to be stored using deduplication, and then the remaining data is compressed.